Tyler Olson
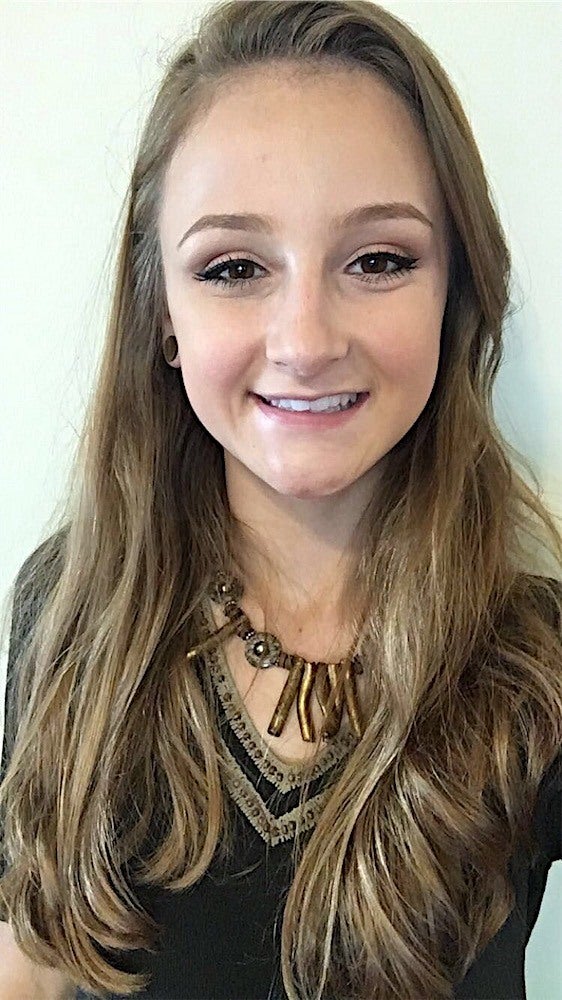
Pronouns: She/Her/Hers
UROP Fellowship: University of Michigan Energy Institute
Research Mentor(s): Brian Ellis, PhD
Civil and Environmental Engineering
Presentation Date: Thursday, July 30, 2020 | Session 2 | Presenter: 5
Authors: Tyler Olson, Ellen Thompson, Kira Tomenchok, Brian Ellis
Abstract
The analysis of core-scale rock properties such as porosity, permeability, and pore size distribution are a vital part in determining the effectiveness of geothermal energy systems. If the rocks that make up a geothermal system are found to be highly reactive under fluid transport, the system may experience unwanted problems (e.g., leakage) that reduce the efficiency of the system. These issues may also hold potential to cause harm to the environment. Because of this, it is imperative that researchers successfully determine the porosity of rocks that may be present in these geothermal systems, as this value is used to predict other important reservoir characteristics (e.g. permeability). X-ray computed tomography (CT) allows for non-destructive imaging of rock cores which can then be used to analyze desired rock properties. There exist both classical and numerical methods that have the ability to determine these fundamental properties. Here, we focus on the improvement of one of the many numerical methods–namely the Weka Segmentation Trainer available on ImageJ software. This software uses operator inputs to inform a machine learning process that differentiates between pore space and mineral space, resulting in a binary image from which the porosity can be readily calculated. CT imaging often results in artefacts such as beam hardening and Gaussian noise; these artefacts can cause the segmentation trainer to misclassify certain regions of the core, resulting in an inaccurate porosity measurement. Through a series of preprocessing filters, these effects can be reduced and the image quality can be improved, ultimately allowing for a more accurate segmentation process. The ideal combination of preprocessing filters is something that has been heavily debated in literature. We propose a workflow consisting of three major filters that are applied prior to segmentation: an unsharp mask filter, bilateral filter, and a beam hardening correction algorithm. By completing a series of segmentation run-throughs with different combinations of these filters and observing the variability in calculated porosity throughout, we are able to test the effectiveness and consistency of our workflow. Then, by using an experimental approach of calculating porosity levels, Mercury Intrusion Porosimetry (MIP), we are able to further test the accuracy of our preprocessing filters and Weka Segmentation Trainer workflow.
Google Slide Presentation
Presentation Script
Research Disciplines
Engineering