Patrick Girard
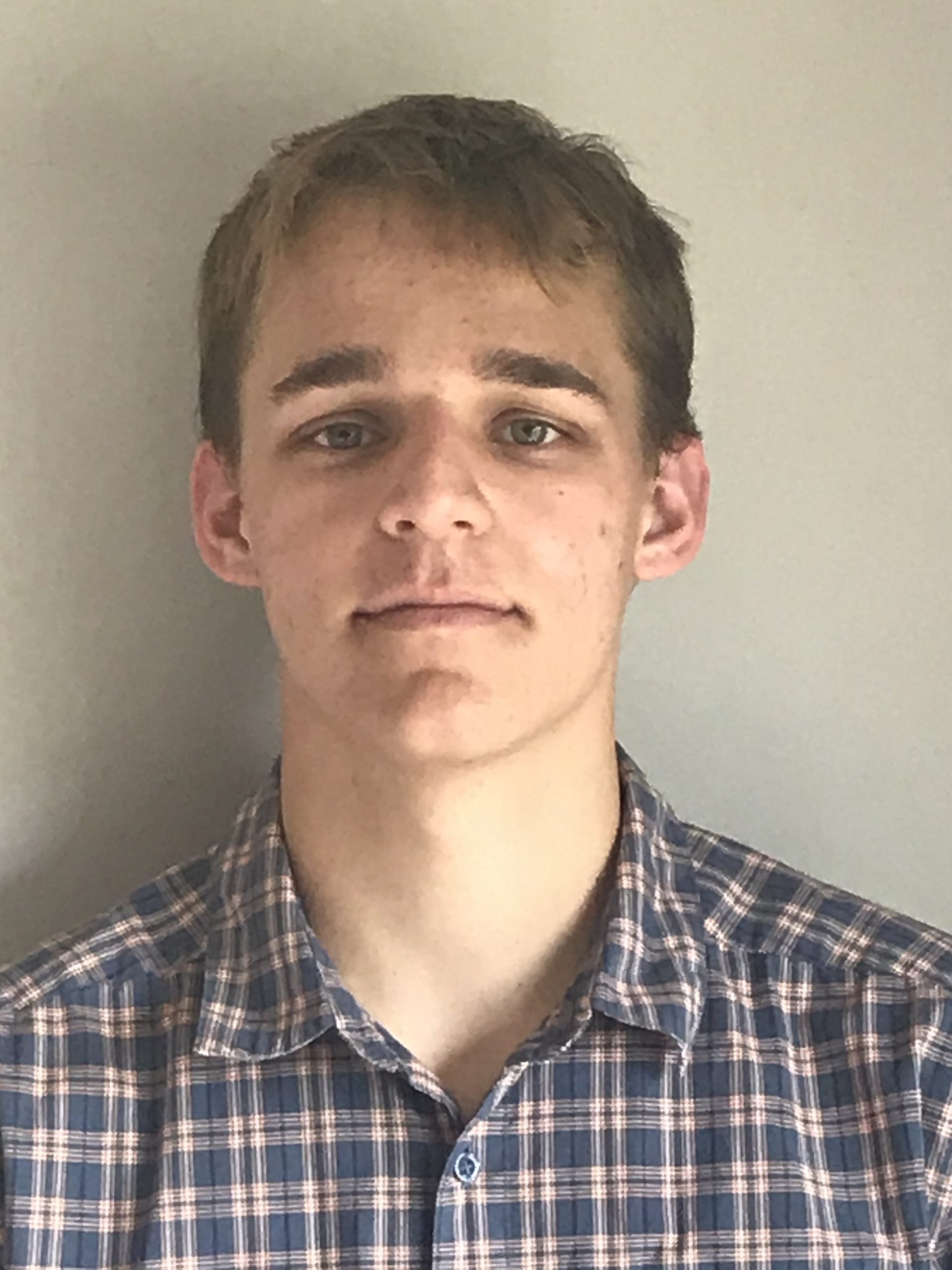
Pronouns: He/Him/His
UROP Fellowship: University of Michigan Energy Institute
Research Mentor(s): Don Siegel, PhD
Department of Mechanical Engineering
Presentation Date: Thursday, July 30, 2020 | Session 1 | Presenter: 8
Authors: Patrick Girard, Steven Kiyabu, Donald Siegel
Abstract
Thermal energy storage (TES) is a potentially advantageous method for storing heat from hours to even months in a “thermal battery”. The methods for TES are sensible, latent and thermochemical. Thermochemical energy storage (TCES) is the most promising due to high energy densities and nearly loss free storage. The main operating principle for TCES technology is a reversible reaction. Salt hydrates have been identified as apt class of material for this technology because of high material energy density, suitable operating temperatures, and use of water as reaction partner. However, more work needs to be done to identify more high-performing salt hydrates. To do this, a graduate student in the Siegel lab, Steven Kiyabu, has performed Density function theory (DFT) simulations for hypothetical and existing salt hydrates that provide us with high quality materials data to explore. This study aims to integrate research at the material and system scale. To do this, an existing TCES prototype using salt hydrates has been identified for further development of a numerical model. This model can help identify high performing salt hydrates in terms of energy density, not just at the materials level but also at the systems level. While this model can categorize materials based on efficiency, energy density, reaction temperatures, and reactor geometry, it is still necessary to look into additional material properties of salt hydrates that affect TES performance. Data for deliquescence relative humidity, which may help determine important factors for reaction kinetics, melting temperature, and entropy of hydration have been gathered for various salts. Existing machine learning scripts with minor modifications are used to detect strong correlations between various data that could provide useful insights. These insights could potentially lead to trends about material properties that could accelerate the identification of high-performing salt hydrates for thermal energy storage.
Google Slide Presentation
Presentation Script
Research Disciplines
Engineering